Artificial intelligence (AI) has emerged as one of the most transformational technologies in contemporary society. AI fundamentally denotes the emulation of human intelligence by machines, especially computer systems, allowing them to execute activities that often necessitate human cognitive abilities. This includes learning, thinking, problem-solving, and decision-making. Artificial intelligence has infiltrated nearly every area, transforming industries by allowing robots to reason and operate in manners once deemed unattainable.
As the technology landscape evolves, AI is becoming an essential tool, improving both personal experiences and commercial operations. This article offers an in-depth examination of artificial intelligence, including its classifications, mechanisms, applications, prospective developments, and ethical implications.
What Is Artificial Intelligence?
Artificial intelligence is an expansive term including various technologies aimed at replicating human intelligence. These technologies rely on computer systems and software that enable machines to execute operations such as experiential learning, pattern recognition, complicated problem-solving, natural language comprehension, and autonomous vehicle operation.
AI represents the intersection of computer science and cognitive science, facilitating the creation of intelligent computers that can make decisions, engage with humans, and comprehend the world through data analysis.
Types of AI
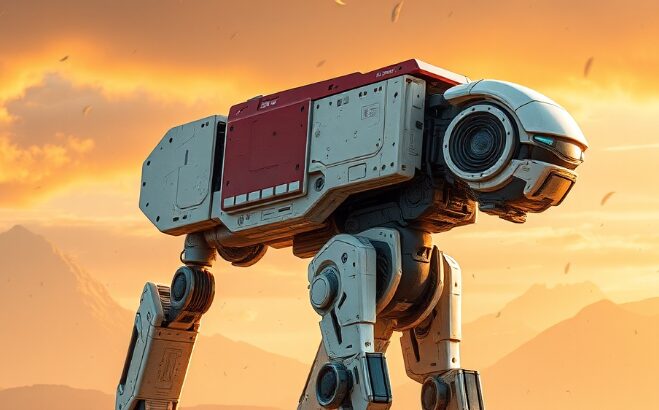
AI can be broadly categorized into different types: Narrow AI and General AI. Each type has distinct capabilities and applications.
1. Narrow AI
Narrow AI, also known as Weak AI, is a type of artificial intelligence system designed to perform specific tasks within a limited scope. These systems excel in their designated area but cannot generalize their knowledge or apply it to unrelated tasks outside of their programming.
Narrow AI is the most common form of AI in use today and powers many everyday technologies such as virtual assistants, recommendation systems, and autonomous driving features in cars. Examples of Narrow AI include Siri, Alexa, Google Assistant, facial recognition systems, streaming services like Netflix and Spotify, autonomous vehicles, and medical diagnostics.
Narrow AI works using machine learning and deep learning techniques, where algorithms are trained on vast amounts of data to identify patterns, make predictions, and optimize outcomes. These systems can improve over time as they are exposed to more data and learn from their experiences, but they remain restricted to their specific domain. For example, a machine learning algorithm trained on images of cats can accurately identify cats in new images but cannot recognize a dog or understand concepts outside of image recognition without being specifically trained for those tasks.
2. General AI
General AI, also known as Strong AI or Artificial General Intelligence (AGI), is a type of artificial intelligence that seeks to imitate human cognitive abilities in a variety of domains. It would be capable of doing specialized tasks, learning, thinking, comprehending complicated concepts, and applying information to novel, unknown situations in a manner equal to human intelligence.
Human-like intelligence, adaptability, consciousness and self-awareness, and domain-wide learning are all key properties of General AI. However, the current state of General AI is primarily theoretical and distant from being implemented. Most AI systems in use today are Narrow AI, which is highly specialized and limited to certain tasks such as image recognition, natural language processing, or self-driving vehicles.
How AI Works
The fundamental mechanics of artificial intelligence are based on algorithms, data processing, and machine learning methodologies. AI systems depend significantly on extensive datasets to train algorithms, enabling the system to enhance its performance progressively. Machine learning and deep learning are essential components of AI system functionality.
1. Machine Learning
Machine Learning (ML) is a branch of artificial intelligence that focuses on creating algorithms and statistical models that allow computers to learn from data and improve their performance on jobs without having to be explicitly programmed for each one. It enables machines to “learn” patterns from data, make decisions and predictions based on that data, and improve their outcomes as they process more information.
Machine learning works by training algorithms on massive datasets, allowing them to detect patterns, predict outcomes, and identify trends. The primary components of this process include data, features, algorithms, models, training, testing, and learning.
ML is classified into 3 types: supervised, unsupervised, and reinforcement learning. Supervised learning is the process of training an algorithm on a labeled dataset so that it can find patterns in the training data and transfer the input to the proper output. Common algorithms include linear regression, logistic regression, support vector machines (SVMs), decision trees, random forests, and neural networks. Unsupervised learning examines data without labeled outcomes to uncover underlying patterns or structures, clustering similar data points together, or lowering data dimensionality for better interpretation.
2. Deep Learning
Deep learning is a kind of machine learning that use artificial neural networks with multiple layers to represent intricate patterns in extensive datasets. It is especially effective for jobs requiring substantial data, such as picture recognition, natural language processing, and speech recognition, where it can autonomously identify complex structures and representations within the data.
Fundamental principles in deep learning encompass neural networks, activation functions, backpropagation, loss functions, and optimization techniques. Neural networks are algorithms modeled after the architecture and operation of the human brain, comprising interconnected neurons. The input layer is the initial layer where data is introduced into the system, succeeded by hidden layers that enable the network to simulate intricate patterns.
Backpropagation is a method by which the network modifies its internal parameters in response to prediction mistakes. The loss function quantifies the discrepancy between the model’s predictions and the actual values, while optimization methods such as Stochastic Gradient Descent (SGD) or more sophisticated variants like Adam are employed to minimize the loss function.
Applications of AI
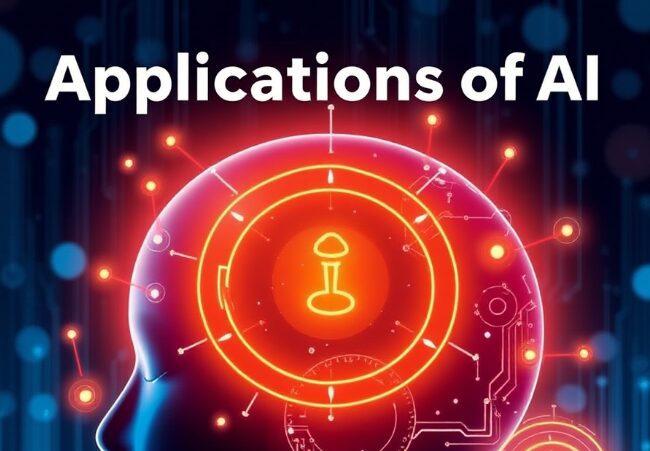
Artificial intelligence is no longer restricted to research laboratories; it is already exerting a significant influence across multiple industries. Artificial intelligence technologies are revolutionizing conventional procedures, enhancing operational efficiency, intelligence, and adaptability to real-world circumstances. Among the most significant applications are:
1. Healthcare
Artificial intelligence is utilized in healthcare to detect illnesses, forecast patient prognoses, and create individualized treatment strategies. Artificial intelligence systems can evaluate medical images, identify anomalies, and aid physicians in rendering precise diagnoses. Moreover, machine learning algorithms are employed to create novel medication regimens and enhance patient care.
The capacity of AI to analyze extensive datasets is transforming medical research. It allows healthcare practitioners to discern patterns in patient data that humans cannot notice, hence promoting early diagnosis and treatment. AI-driven systems such as IBM Watson are currently employed to aid oncologists in cancer diagnosis and treatment recommendation.
2. Finance
AI has become a cornerstone of the financial industry. It is used to detect fraudulent activities, optimize investment portfolios, and provide personalized financial advice to customers. AI-powered algorithms analyze historical data and market trends to offer real-time insights and make trading decisions with minimal human intervention.
Banks and financial institutions also use AI to improve customer service through chatbots, automate risk assessment, and enhance the accuracy of credit scoring models. In fraud detection, AI systems can identify unusual patterns in transactions, alerting organizations to potential threats before they cause significant damage.
3. Customer Service
Automated customer service systems, such as chatbots and virtual assistants, rely on AI to interact with customers and address their needs. These systems can answer frequently asked questions, resolve issues, and even recommend products or services based on customer preferences.
AI enhances the efficiency and effectiveness of customer service operations by providing quick, accurate responses, reducing wait times, and freeing up human agents to handle more complex tasks. Virtual assistants, such as Amazon’s Alexa and Google’s Assistant, are becoming increasingly sophisticated in their ability to understand natural language and carry out a wide range of tasks.
4. Manufacturing
In manufacturing, AI is optimizing production processes, improving quality control, and reducing costs. AI-powered robots and automation systems can handle repetitive tasks with high precision, increasing overall efficiency. Predictive maintenance systems use AI to monitor machinery and detect potential failures before they occur, minimizing downtime.
AI is also being used in supply chain management to optimize logistics, forecast demand, and improve inventory control. By analyzing data from various sources, AI systems can make real-time decisions that enhance operational efficiency and reduce waste.
5. Transportation
One of the most exciting applications of AI in transportation is the development of self-driving cars. Autonomous vehicles use AI to navigate roads, recognize obstacles, and make real-time driving decisions. Companies like NVIDIA, Tesla, Waymo, and Uber are at the forefront of this technology, working to create safer and more efficient transportation systems.
AI is also being used to improve traffic management, reduce congestion, and enhance public transportation services. By analyzing traffic patterns and predicting demand, AI systems can optimize routes and schedules, leading to more efficient and sustainable transportation networks.
Conclusion
AI is rapidly transforming our daily lives. From healthcare to transportation, AI is enabling new possibilities and improving efficiency across industries. While the potential benefits of AI are immense, it is crucial to address the ethical challenges that come with this technology. By developing and deploying AI responsibly, we can harness its power to solve some of the most pressing global issues and create a better future for all.